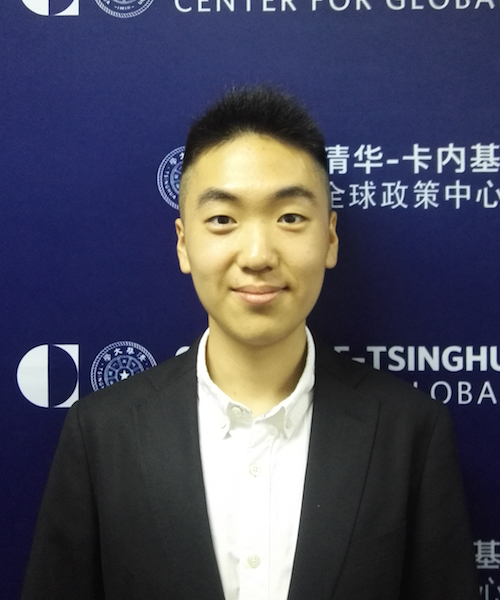
Addressing Global Issues with Innovations of the Digital Age —A Case on Combating Internet Terrorism
Chenyu Wu | June 3, 2018
Responding To: Living in a Digital Age: How Young Chinese and Americans Can Employ Innovation to Resolve Global Issues
Jessie Dalman
The term “artificial intelligence,” or AI, was first coined at a conference at Dartmouth College in 1956 to describe the notion that “every aspect of learning or any other feature of intelligence can… be so precisely described that a machine can be made to simulate it.” The United States has largely remained at the forefront of AI development since, pioneering a subfield of study known as “machine learning,” which refers to processes by which algorithms autonomously become ‘smarter’ or more accurate as they are exposed to more data.
In recent years, Chinese companies have invested more in artificial intelligence development. Machine learning relies upon the collection and analysis of massive data sets, and China, “whose 1.38 billion people makes it the world’s biggest user base and data pool, is a ‘paradise’ for machine learning technology.” Furthermore, large technology companies, such as the e-commerce giant Alibaba, are rapidly increasing China’s competitive edge in the digital space. As articulated by Richard Waters in the Financial Times, “China’s vast markets, along with the emergence of a group of internet leaders… should provide plenty of raw material to fuel the rise of intelligent systems.”
In short, AI has developed to become a tangible reality in our world, packed with massive potential. Along with this potential, however, come considerable responsibilities. Both the United States and China can significantly influence the future of machine learning by identifying and addressing algorithmic biases.
To understand algorithmic bias, consider the use of algorithms in predictive modeling, wherein data is used to predict outcomes. Machine learning vastly strengthens predictive models by developing “the best prediction for cases where [values] are missing.” In this way, “machine learning is able to manage vast amounts of data and detect many more complex patterns within them, often attaining superior predictive power.”
Algorithmic bias manifests when algorithms are written to reflect existing prejudices in our imperfect world. An algorithm designed to predict the likelihood of recidivism for criminal offenders, for instance, might unconsciously be written to assign higher risk assessment scores to African Americans. Mathematician Cathy O’Neil explains these disparities by postulating that people often place too much trust in models, because they believe that math can inherently remove human biases. “[Algorithms] replace human processes, but they’re not held to the same standards,” she says. In other words, “algorithmic bias is one of the biggest risks because it compromises the very purpose of machine learning. This often-overlooked defect can trigger costly errors and, left unchecked, can pull projects and organizations into entirely wrong directions.”
Alibaba and Alphabet, two of the world’s largest technology companies located in China and the United States, respectively, frequently confront accusations of algorithmic bias. In 2017, Alphabet acknowledged that latent bias in its algorithms could affect Google search results. When prompted to search for the word ‘physicist,’ for example, a machine might review photos of past physicists, a majority of whom have been men. Consequently, the algorithm could conclude that only men can be physicists, thus introducing a latent bias against women into the search process. Shortly thereafter, Alphabet announced the creation of an ethics research unit within its AI division, a subsidiary known as DeepMind, to investigate and combat similar biases.
Alibaba, however, has yet to address similar concerns. Speaking at the World Economic Forum in Davos, Switzerland in January 2018, Alibaba CEO Jack Ma noted that AI might impose a negative impact by “[killing] many jobs,” but did not mention the potential for implicit bias in big data. Indeed, as noted by an article in the MIT technology review, “... unlike its U.S. counterparts, Alibaba isn’t involved with ethics groups… and unlike DeepMind… it doesn’t have an internal ethics division.” Though China is poised to be a major player in the machine learning revolution, Chinese companies at the forefront of this movement do not feel compelled to contend with the issue of algorithmic bias and its potential impact on society.
American and Chinese companies need to work together to correct this ethical oversight. Alphabet, for instance, could help Alibaba construct an internal ethics division similar to the DeepMind research unit. Furthermore, engineers in both countries should together write a code of ethics for machine learning practice. This collaboration would prevent the values of one country from being imposed upon the other, and ensure that all major stakeholders are able to voice their opinions, leading to greater probability of future adherence to the agreed upon standards. By taking ethics seriously, China and the United States can harness the power of machine learning to truly create a better world.
Jessie Dalman is a senior at Stanford University majoring in history with a concentration in conflict studies.
Chenyu Wu | June 3, 2018
Yunxin Wang | June 1, 2018
Michael Mullaney | May 30, 2018
Hongjin Xu | May 29, 2018
Cynthia Wang | May 28, 2018
Ruolin Zhao | May 27, 2018
Yamillet Payano | May 24, 2018
Haile Chen | May 22, 2018